UD professor and collaborator mine literature, electronic health records for connections between HIV and substance abuse
More than 36 million people worldwide live with HIV, 1.2 million in the United States alone. While the lifespan of those living with HIV has significantly improved thanks to antiretroviral therapies, individuals with HIV still face challenges.
One big quality-of-life concern is that individuals with HIV are more likely than their peers without the virus to suffer from substance abuse disorders (SUD).
Why this occurs is unknown. Ilya Safro, associate professor of computer and information sciences at the University of Delaware, is using artificial intelligence (AI) and big data to analyze biomedical and health records for connections that might explain the link between HIV infection, HIV-associated dementia and substance abuse comorbidity. The work, funded by a $2.1 million grant from the National Institutes of Health, is a collaborative effort with Michael Shtutman, associate professor of drug discovery and biomedical sciences at the University of South Carolina.
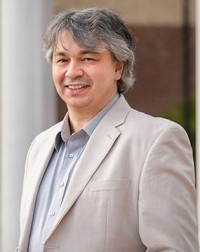
Prof. Ilya Safro
Safro, who joined the UD faculty in 2021, has expertise in developing algorithms and artificial intelligence systems, with a focus on natural language processing and something called scientific hypothesis generation.
Hypothesis generation involves mining various data sets to create a large knowledge system that can be queried, and then using machine learning algorithms to establish a logical connection between things that might otherwise seem unrelated. For example, in health care, hypothesis generation could be used to take an educated guess at the connections between diseases and genes or proteins, or how a medication is related to a side effect, which might also be related to something else.
“When researchers read scientific literature, they are trying to establish direct connections or complex, logical patterns between things that have not been known to be connected,” Safro said. “In my lab, we develop advanced machine learning pipelines that establish such unknown connections using automated analysis of biomedical literature and by combining scientific literature with other data sources.”
These data sources could include, for example, descriptions of chemicals, molecules used in drugs and reports from clinical trials. “We process all of them into a huge single knowledge discovery system, and then ask it to discover novel connections,” Safro said.
In the 1980s, this approach led to what is now an established connection between the benefits of fish oil for some people who have Raynaud’s disease. Raynaud’s disease is a condition where some areas of a person’s body, such as fingers and toes, periodically feel cold due to a constriction of small arteries that supply blood to the skin. Previously, no direct link between Raynaud’s and fish oil existed in the literature. Researchers were able to establish one, however, by mining the titles of published research and detecting indirect mentions of both topics in some of the paper titles. This led to medical experiments that confirmed fish oil improves cold tolerance in folks with primary Raynaud’s.
Today, Safro’s sophisticated mathematical methods can connect the dots between more than just first-layer, indirect connections. But how is this information useful? Put simply, medical research is expensive and risky. In an ideal world, it would be nice to have a front-end system to identify possible connections between things like diseases, genes, available treatments and more before pursuing costly experiments, recalls, side effects discovery, and so on.
According to Safro, PubMed and PubMed Central are readily available resources that contain over 33 million abstracts and 7.8 million full text scientific papers from over 5,500 journals in 60 languages. Close to 900,0000 papers are added to PubMed each year, he said, creating a constantly growing data set. The National Library of Medicine, meanwhile, contains other data sets on gene expression, chemicals, biosystems, clinical trials and more. Then there is proprietary information, such as electronic health records, lab journals, electronic notebooks.
With this new funding, Safro and Shtutman are exploring new treatments for HIV-associated neurocognitive disorder. Approximately 40% of people living with HIV over age 60 develop neurocognitive impairments, including severe dementia. There is no current treatment for HIV-associated neurocognitive disorder. The development of new drugs is time consuming and costly; therefore, Shtutman and Safro groups are focusing on repurposing the existing approved drugs to treat the disease.
In previously published work, Safro and colleagues used natural language processing and AI methods to establish possible connections between genes and HIV-associated dementia.
Now the collaborating scientists are going one layer deeper — Safro and his collaborators plan to combine their AI system’s capabilities with electronic health record data from the U.S. Department of Veterans Affairs to explore whether there are connections between HIV, HIV-associated dementia, and certain types of drug abuse to look for possible solutions.
It’s a multifaceted problem, one designed to establish deeper connections to the factors at play that can result in someone with HIV ending up also being a drug abuser. And in this case, the HIV-associated dementia may be a co-mitigating factor for HIV and substance abuse.
The process will be iterative, too. Safro’s team will focus on advanced machine learning methods and algorithms to explore health records of HIV and substance abuse disorder patients, to develop a system for analyzing VA data and generating hypotheses for potential medications that may warrant experimental validation and further clinical development.
“Let’s say the VA’s statistical analysis suggests that a particular combination of the diseases and drug abuse is extremely important to study,” Safro said. “They will give this information to us, and we will try to run it versus all possible drugs and see what drugs can treat this combination of diseases.”
The overarching goal is to use AI and machine learning to read and analyze scientific publications, biomedical datasets and electronic health records to understand the connection and to discover whether there are existing drugs in the market that can be repurposed to manage this combination of diseases.
Safro called the interdisciplinary project an excellent opportunity to train the next generation of AI scientists. As part of the project, Ilya Tyagin and Ankit Kulshrestha, UD doctoral students in the Safro lab, are advancing state-of-the-art approaches in automated knowledge discovery and developing scalable algorithmic solutions using UD’s high-performance computing infrastructure. Krish Matta, a high school student from the Charter School of Wilmington, working in Safro’s lab, has also contributed to this project.
While the current project focuses on HIV and substance abuse, Safro said his team’s natural language processing system can make other connections, too. For example, a separate version of this system is being used to understand connections about COVID-19 research, with funding from the National Science Foundation RAPID program.
“We’re looking for ways to create shortcuts that get us to the same end goal while saving time, saving money, and making solutions available for real people faster,” said Safro.
Article by Karen B. Roberts | Graphic illustration by Jeffrey C. Chase with HIV capsid image courtesy Juan Perilla | April 20, 2022